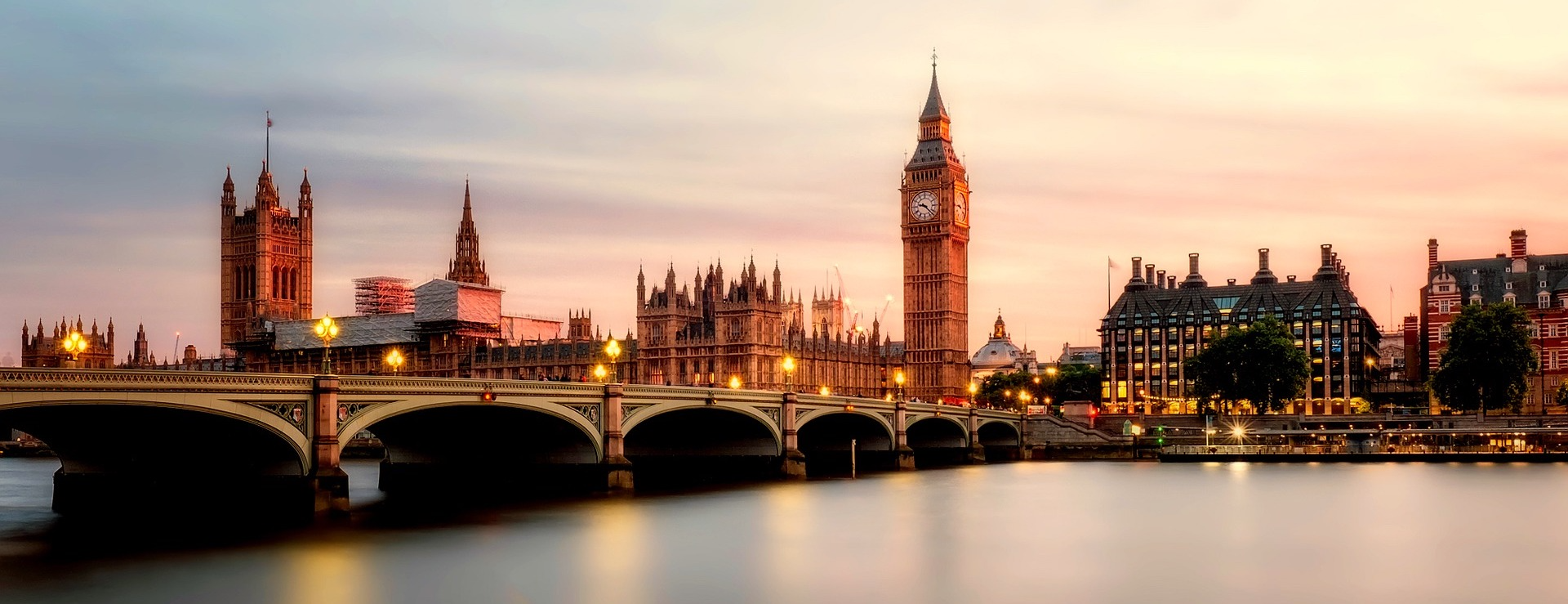
ALA 2021
3 & 4 May 2021, London (Virtual)
News
- The ALA 2021 live sessions will take place via Zoom. The links to the live sessions are available on the AAMAS Workshops and Tutorials Underline platform.
- 27 April 2021: The ALA 2021 papers and video presentations are now available!
- 26 April 2021: The schedule for the ALA 2021 live events is now online! Check out the program for all the details.
- 15 April 2021: We are excited to announce the invited tutorial talk for this year, by Matthew Gombolay!
- 14 April 2021: We invite all authors and participants to join our Slack workspace! Check out the program for more details.
- 8 April 2021: The list of accepted papers is now online.
- 22 March 2021: We are happy to announce our invited speakers for this year, Marc G. Bellemare and Naomi Ehrich Leonard!
- 25 February 2021: Submissions are now closed. We received 62 submissions this year!
- 5 February 2021: The submission deadline has been extended to 24 February 2021 23:59 UTC!
- 5 January 2021: Program Committee members added
- 23 November 2020: ALA 2021 site launched
ALA 2021 - Workshop at AAMAS 2021
Adaptive and Learning Agents (ALA) encompasses diverse fields such as Computer Science, Software Engineering, Biology, as well as Cognitive and Social Sciences. The ALA workshop will focus on agents and multiagent systems which employ learning or adaptation.
This workshop is a continuation of the long running AAMAS series of workshops on adaptive agents, now in its thirteenth year. Previous editions of this workshop may be found at the following urls:
- ALA-20
- ALA-19
- ALA-18
- ALA-17
- ALA-16
- ALA-15
- ALA-14
- ALA-13
- ALA-12
- ALA-11
- ALA-10
- ALA-09
- ALAMAS+ALAg-08
- ALAg-07
- Earlier editions
The goal of this workshop is to increase awareness of and interest in adaptive agent research, encourage collaboration and give a representative overview of current research in the area of adaptive and learning agents and multi-agent systems. It aims at bringing together not only scientists from different areas of computer science (e.g. agent architectures, reinforcement learning, evolutionary algorithms) but also from different fields studying similar concepts (e.g. game theory, bio-inspired control, mechanism design).
The workshop will serve as an inclusive forum for the discussion of ongoing or completed work covering both theoretical and practical aspects of adaptive and learning agents and multi-agent systems.
This workshop will focus on all aspects of adaptive and learning agents and multi-agent systems with a particular amphasis on how to modify established learning techniques and/or create new learning paradigms to address the many challenges presented by complex real-world problems. The topics of interest include but are not limited to:
- Novel combinations of reinforcement and supervised learning approaches
- Integrated learning approaches that work with other agent reasoning modules like negotiation, trust models, coordination, etc.
- Supervised multi-agent learning
- Reinforcement learning (single- and multi-agent)
- Novel deep learning approaches for adaptive single- and multi-agent systems
- Multi-objective optimisation in single- and multi-agent systems
- Planning (single- and multi-agent)
- Reasoning (single- and multi-agent)
- Distributed learning
- Adaptation and learning in dynamic environments
- Evolution of agents in complex environments
- Co-evolution of agents in a multi-agent setting
- Cooperative exploration and learning to cooperate and collaborate
- Learning trust and reputation
- Communication restrictions and their impact on multi-agent coordination
- Design of reward structure and fitness measures for coordination
- Scaling learning techniques to large systems of learning and adaptive agents
- Emergent behaviour in adaptive multi-agent systems
- Game theoretical analysis of adaptive multi-agent systems
- Neuro-control in multi-agent systems
- Bio-inspired multi-agent systems
- Applications of adaptive and learning agents and multi-agent systems to real world complex systems
Extended and revised versions of papers presented at the workshop will be eligible for inclusion in a journal special issue (see below).
Important Dates
Submission Details
Papers can be submitted through EasyChair.
We invite submission of original work, up to 8 pages in length (excluding references) in the ACM proceedings format (i.e. following the AAMAS formatting instructions). This includes work that has been accepted as a poster/extended abstract at AAMAS 2021. Additionally, we welcome submission of preliminary results, i.e. work-in-progress, as well as visionary outlook papers that lay out directions for future research in a specific area, both up to 6 pages in length, although shorter papers are very much welcome, and will not be judged differently. Finally, we also accept recently published journal papers in the form of a 2 page abstract.
Furthermore, for submissions that were rejected or accepted as extended abstracts at AAMAS, we encourage authors to also append the received reviews. This is simply a recommendation and it is optional. Authors can also include a short note or changelist they carried out on the paper. The reviews can be appended at the end of the submission file and do not count towards the page limit.
All submissions will be peer-reviewed (single-blind). Accepted work will be allocated time for poster and possibly oral presentation during the workshop. Extended versions of original papers presented at the workshop will also be eligible for inclusion in a post-proceedings journal special issue.
Journal Special Issue
We are delighted to announce that extended versions of all original contributions at ALA 2021 will be eligible for inclusion in a special issue of the Springer journal Neural Computing and Applications (Impact Factor 4.213). The deadline for submitting extended papers is 15 September 2021 22 November 2021.
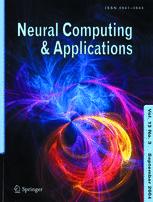
We will post further details about the submission process and expected publication timeline after the workshop.
Program
Except for the invited talks, ALA 2021 will take place in an asynchronous manner. In order to facilitate discussions over all the contributions as well as social interactions, we invite all authors and participants to join our Slack workspace.
In order to organise discussions, we ask authors/participants to create channels with the name paper-#. We have added below a unique number for each contribution.
The ALA 2021 live sessions will take place via Zoom. The links to the live sessions are available on the AAMAS Workshops and Tutorials Underline platform. In order to access the platform, registration is required and it is free.
Monday, 3 May
14:50 - 15:00 BST | Welcome & Opening Remarks |
15:00 - 16:00 BST | Discussion Panel Topic: Links between Industry, Academia and Governments in AI development Chair: Matthew Gombolay (Georgia Institute of Technology) Panelists:
|
16:00 - 17:00 BST | Invited Talk: Naomi Ehrich Leonard Opinion Dynamics and Reinforcement Learning in Multi-agent Games |
Tuesday, 4 May
15:00 - 16:00 BST | Invited Talk: Marc G. Bellemare Autonomous navigation of superpressure balloons using reinforcement learning |
17:00 - 18:00 BST | Invited Tutorial: Matthew Gombolay Interpretable Models for Interactive Multi-Robot Learning |
18:00 - 18:15 BST | Awards, closing remarks and ALA 2022
Best Paper Award:
Jacopo Castellini, Sam Devlin, Frans A. Oliehoek and Rahul Savani, Difference Rewards Policy Gradients |
Accepted Papers
Long Talks
Paper # | Details | Title | Authors |
---|---|---|---|
5 | [Paper][Video][Slides] | Guaranteeing the Learning of Ethical Behaviour through Multi-Objective Reinforcement Learning | Manel Rodríguez-Soto, Juan Antonio Rodriguez Aguilar and Maite Lopez-Sanchez |
9 | [Paper][Video][Slides] | Difference Rewards Policy Gradients | Jacopo Castellini, Sam Devlin, Frans A. Oliehoek and Rahul Savani |
11 | [Paper][Video][Slides] | A Multi-Arm Bandit Approach To Subset Selection Under Constraints | Ayush Deva, Kumar Abhishek and Sujit Gujar |
24 | [Paper][Video][Slides] | Self-Transfer Reinforcement Learning | Hélène Plisnier, Denis Steckelmacher and Ann Nowé |
26 | [Paper][Video][Slides] | Sample-Efficient Reinforcement Learning for Continuous Actions with Continuous BDPI | Denis Steckelmacher, Hélène Plisnier and Ann Nowe |
27 | [Paper][Video][Slides] | Combining Off and On-Policy Training in Model-Based Reinforcement Learning | Alexandre Borges and Arlindo Oliveira |
28 | [Paper][Video][Slides] | The Effect of Q-function Reuse on the Total Regret of Tabular, Model-Free, Reinforcement Learning | Volodymyr Tkachuk, Sriram G. Subramanian and Matthew E. Taylor |
30 | [Paper][Video][Slides] | Towards Open Ad Hoc Teamwork Using Graph-based Policy Learning | Muhammad Arrasy Rahman, Niklas Hopner, Filippos Christianos and Stefano V. Albrecht |
33 | [Paper][Video][Slides] | Risk-Aware and Multi-Objective Decision Making with Distributional Monte Carlo Tree Search | Conor F Hayes, Mathieu Reymond, Diederik M. Roijers, Enda Howley and Patrick Mannion |
39 | [Paper][Video][Slides] | Evolutionary Game Theory Squared: Evolving Agents in Endogenously Evolving Zero-Sum Games | Stratis Skoulakis, Tanner Fiez, Ryann Sim, Georgios Piliouras and Lillian Ratliff |
40 | [Paper][Video][Slides] | Temporal Difference and Return Optimism in Cooperative Multi-Agent Reinforcement Learning | Mark Rowland, Shayegan Omidshafiei, Daniel Hennes, Will Dabney, Andrew Jaegle, Paul Muller, Julien Perolat and Karl Tuyls |
44 | [Paper][Video][Slides] | Comparative Evaluation of Cooperative Multi-Agent Deep Reinforcement Learning Algorithms | Georgios Papoudakis, Filippos Christianos, Lukas Schäfer and Stefano Albrecht |
Short Talks
Paper # | Details | Title | Authors |
---|---|---|---|
8 | [Paper][Video][Slides] | Is the Cerebellum a Model-Based Reinforcement Learning Agent? | Bharath Masetty, Reuth Mirsky, Ashish Deshpande, Michael Mauk and Peter Stone |
14 | [Paper][Video][Slides] | Exploring the Impact of Tunable Agents in Sequential Social Dilemmas | David O'Callaghan and Patrick Mannion |
18 | [Paper][Video][Slides] | Differential Privacy Meets Maximum-weight Matching | Panayiotis Danassis, Aleksei Triastcyn and Boi Faltings |
20 | [Paper][Video][Slides] | Communication Strategies in Multi-Objective Normal-Form Games | Willem Röpke, Roxana Rădulescu, Diederik Roijers and Ann Nowé |
29 | [Paper][Video][Slides] | Robustness to Adversarial Attacks in Learning-Enabled Controllers | Zikang Xiong, Joe Eappen, He Zhu and Suresh Jagannathan |
34 | [Paper][Video][Slides] | Scaling Multi-Agent Reinforcement Learning with Selective Parameter Sharing | Filippos Christianos, Georgios Papoudakis, Muhammad Arrasy Rahman and Stefano Albrecht |
36 | [Paper][Video][Slides] | Knowledge Infused Policy Gradients with Upper Confidence Bound for Relational Bandits | Kaushik Roy, Qi Zhang, Manas Gaur and Amit Sheth |
38 | [Paper][Video][Slides] | Online Learning in Periodic Zero-Sum Games: von Neumann vs Poincare | Tanner Fiez, Ryann Sim, Stratis Skoulakis, Georgios Piliouras and Lillian Ratliff |
43 | [Paper][Video][Slides] | Dominance Criteria and Solution Sets for the Expected Scalarised Returns | Conor F Hayes, Timothy Verstraeten, Diederik M. Roijers, Enda Howley and Patrick Mannion |
48 | [Paper][Video][Slides] | Semi-On-Policy Training for Sample Efficient Multi-Agent Policy Gradients | Bozhidar Vasilev, Tarun Gupta, Bei Peng and Shimon Whiteson |
49 | [Paper][Video][Slides] | Particle Value Functions in Imperfect Information Games | Michal Sustr, Vojtech Kovarik and Viliam Lisy |
53 | [Paper][Video][Slides] | Value-Based Reinforcement Learning for Sequence-to-Sequence Models | Fabian Retkowski |
55 | [Paper][Video][Slides] | LTLf-based Reward Shaping for Reinforcement Learning | Mahmoud Elbarbari, Kyriakos Efthymiadis, Bram Vanderborght and Ann Nowé |
58 | [Paper][Video][Slides] | Truly Black-box Attack on Reinforcement Learning via Environment Poisoning | Hang Xu and Rabinovich Zinovi |
62 | [Paper][Video][Slides] | Data-Driven Reinforcement Learning for Virtual Character Animation Control | Vihanga Gamage, Cathy Ennis and Robert Ross |
Spotlight
Paper # | Details | Title | Authors |
---|---|---|---|
4 | [Paper][Video][Slides] | A Second-Order Adaptive Cognitive Agent Model for Emotion Regulation in Addictive Social Media Behaviour | Elisabeth Fokker, Xinran Zong and Jan Treur |
6 | [Paper][Video][Slides] | RLupus: Cooperation through Emergent Communication in The Werewolf Social Deduction Game | Nicolo Brandizzi, Luca Iocchi and Davide Grossi |
16 | [Paper][Video][Slides] | Reward-Sharing Relational Networks in Multi-Agent Reinforcement Learning as a Framework for Emergent Behavior | Hossein Haeri, Reza Ahmadzadeh and Kshitij Jerath |
17 | [Paper][Video][Slides] | Predicting Aircraft Trajectories via Imitation Learning | Theocharis Kravaris, Alevizos Bastas and George Vouros |
19 | [Paper][Video][Slides] | LIEF: Learning to Influence through Evaluative Feedback | Ramona Merhej and Mohamed Chetouani |
21 | [Paper][Video][Slides] | Adaptive learning for financial markets mixing model-based and model-free RL | Eric Benhamou, David Saltiel, Serge Tabachnik, Sui Kai Wong and François Chareyron |
23 | [Paper][Video][Slides] | Reasoning about Human Behavior in Ad-Hoc Teamwork | Jennifer Suriadinata, William Macke, Reuth Mirsky and Peter Stone |
25 | [Paper][Video][Slides] | Robust Online Planning with Imperfect Models | Maxim Rostov and Michael Kaisers |
31 | [Paper][Video][Slides] | Fast Approximate Solutions using Reinforcement Learning for Dynamic Capacitated Vehicle Routing with Time Windows | Nazneen N Sultana, Vinita Baniwal, Ansuma Basumatary, Piyush Mittal, Supratim Ghosh and Harshad Khadilkar |
35 | [Paper][Video][Slides] | HAMMER: Multi-Level Coordination of Reinforcement Learning Agents via Learned Messaging | Nikunj Gupta, Gopalakrishnan Srinivasaraghavan, Swarup Mohalik, Nishant Kumar and Matthew E. Taylor |
37 | [Paper][Video][Slides] | Work-in-progress: Comparing Feedback Distributions in Limited Teacher-Student Settings | Calarina Muslimani, Kerrick Johnstonbaugh and Matthew Taylor |
41 | [Paper][Video][Slides] | Searching with Opponent-Awareness | Timy Phan |
45 | [Paper][Video][Slides] | Deep reinforcement learning for rehabilitation planning of water pipes network | Zaharah A. Bukhsh, Nils Jansen and Hajo Molegraaf |
50 | [Paper][Video][Slides] | Latent Property State Abstraction For Reinforcement learning | John Burden, Daniel Kudenko and Sajjad Kamali Siahroudi |
51 | [Paper][Video][Slides] | Work-in-progress: Revisiting Experience Replay in Non-stationary Environments | Derek Li, Andrew Jacobsen and Adam White |
52 | [Paper][Video][Slides] | Evaluating household-pooled universal testing to control COVID-19 epidemics, using an individual-based model | Pieter Libin, Lander Willen, Timothy Verstraeten, Andrea Torneri, Joris Vanderlocht and Niel Hens |
57 | [Paper][Video][Slides] | Graph Learning based Generation of Abstractions for Reinforcement Learning | Yuan Xue, Daniel Kudenko and Megha Khosla |
61 | [Paper][Video][Slides] | NovelGridworlds: A Benchmark Environment for Detecting and Adapting to Novelties in Open Worlds | Shivam Goel, Gyan Tatiya, Matthias Scheutz and Jivko Sinapov |
Invited Talks
Marc G. Bellemare
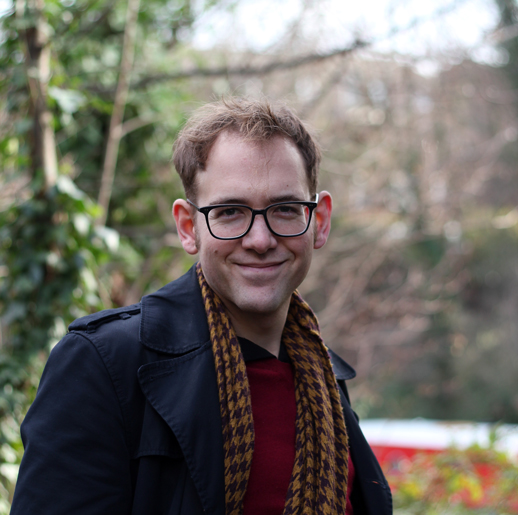
Affiliations:
Research Scientist, Google Research (Brain team)
Adjunct Professor, McGill University
Canada CIFAR AI Chair, Mila
Website: http://www.marcgbellemare.info
Talk Title: Autonomous navigation of superpressure balloons using reinforcement learning
Abstract: Efficiently navigating a superpressure balloon in the stratosphere requires the integration of a multitude of cues, such as wind speed and solar elevation, and the process is complicated by forecast errors and sparse wind measurements. Coupled with the need to make decisions in real time, these factors rule out the use of conventional control techniques. This talk describes the use of reinforcement learning to create a high-performing flight controller for Loon superpressure balloons. Our algorithm uses data augmentation and a self-correcting design to overcome the key technical challenge of reinforcement learning from imperfect data, which has proved to be a major obstacle to its application to physical systems. We deployed our controller to station Loon balloons at multiple locations across the globe, including a 39-day controlled experiment over the Pacific Ocean. Analyses show that the controller outperforms Loon’s previous algorithm and is robust to the natural diversity in stratospheric winds. These results demonstrate that reinforcement learning is an effective solution to real-world autonomous control problems in which neither conventional methods nor human intervention suffice, offering clues about what may be needed to create artificially intelligent agents that continuously interact with real, dynamic environments.
Bio: Marc G. Bellemare leads the reinforcement learning efforts at Google Research in Montreal and holds a Canada CIFAR AI Chair at the Quebec Artificial Intelligence Institute (Mila). He received his Ph.D. from the University of Alberta, where he developed the highly-successful Arcade Learning Environment benchmark. From 2013 to 2017 he held the position of research scientist at DeepMind in London, UK, where he made major contributions to deep reinforcement learning, in particular pioneering the distributional method. Marc G. Bellemare is also a CIFAR Learning in Machines & Brains Fellow and an adjunct professor at McGill University.
Naomi Ehrich Leonard
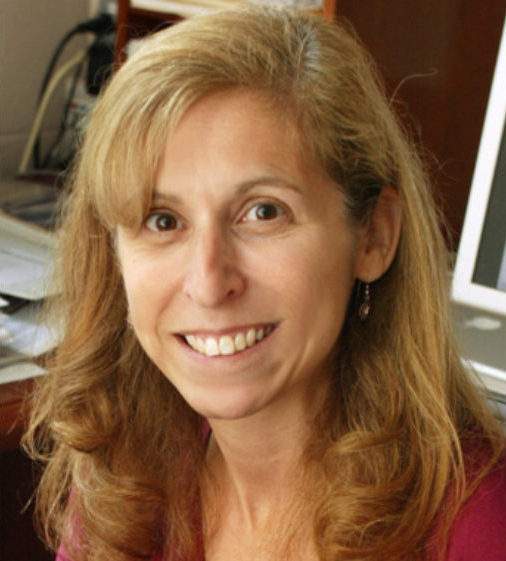
Affiliation: Princeton University
Website: https://naomi.princeton.edu
Talk Title: Opinion Dynamics and Reinforcement Learning in Multi-agent Games
Abstract: I will discuss a recently proposed model of opinion dynamics and its use, as a model of reinforcement learning with distributed information sharing, in the study of multi-agent strategic interaction. Our model describes continuous-time opinion dynamics for an arbitrary number of agents that communicate over a network and form real-valued opinions about an arbitrary number of options. Many models in the literature update agent opinions using a weighted average of their neighbors’ opinions. Our model generalizes these models by applying a sigmoidal saturation function to opinion exchanges. This makes the update fundamentally nonlinear: opinions form through a bifurcation yielding multi-stability of network opinion configurations. Leveraging idealized symmetries in the system, qualitative behavioral regimes can be distinguished explicitly in terms of just a few parameters. I will show how to interpret the opinion dynamics as a model of reinforcement learning in multi-agent finite games and discuss implications for games including the prisoner’s dilemma and the traveler’s dilemma. This is joint work with Anastasia Bizyaeva, Alessio Franci, Shinkyu Park, and Yunxiu Zhou, and based on papers https://arxiv.org/abs/2009.04332v2 and https://arxiv.org/abs/2103.14764.
Bio: Naomi Ehrich Leonard is the Edwin S. Wilsey Professor of Mechanical and Aerospace Engineering and associated faculty member of the Program in Applied and Computational Mathematics at Princeton University. She is Director of Princeton's Council on Science and Technology and affiliated faculty member of the Princeton Neuroscience Institute and Program on Quantitative and Computational Biology. She received her BSE in Mechanical Engineering from Princeton University and her PhD in Electrical Engineering from the University of Maryland. Leonard is a MacArthur Fellow, a member of the American Academy of Arts and Sciences, and a Fellow of the ASME, IEEE, IFAC, and SIAM. Prof. Leonard's background includes feedback control theory, nonlinear dynamics, geometric mechanics, and robotics, where she has made contributions both to theory and to application. She studies and designs complex, dynamical systems comprised of many interacting agents, including, for example, animais, robots, and/or humans that move, sense, and decide together. Her research program emphasizes the development of analytically tractable mathematical models of collective dynamics that provide the systematic means to examine the role of feedback (responsive behavior), network interconnection (who is communicating with whom), heterogeneity (individual differences) in the behavior, learning, and resilience of groups in changing environments.
Matthew Gombolay
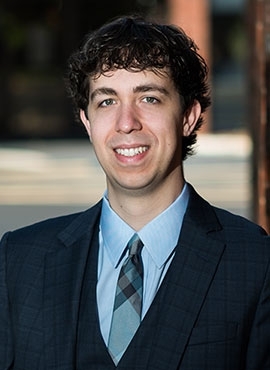
Affiliation: Georgia Institute of Technology
Website: https://core-robotics.gatech.edu/people/matthew-gombolay/
Talk Title: Interpretable Models for Interactive Multi-Robot Learning
Bio: Dr. Matthew Gombolay is an Assistant Professor of Interactive Computing at the Georgia Institute of Technology. He received a B.S. in Mechanical Engineering from the Johns Hopkins University in 2011, an S.M. in Aeronautics and Astronautics from MIT in 2013, and a Ph.D. in Autonomous Systems from MIT in 2017. Gombolay’s research interests span robotics, AI/ML, human-robot interaction, and operations research. Between defending his dissertation and joining the faculty at Georgia Tech, Dr. Gombolay served as technical staff at MIT Lincoln Laboratory, transitioning his research to the U.S. Navy and earning a R&D 100 Award. His publication record includes a best paper award from the American Institute for Aeronautics and Astronautics, a finalist for best student paper at the American Controls Conference, and a finalist for best paper at the Conference on Robot Learning. Dr Gombolay was selected as a DARPA Riser in 2018, received 1st place for the Early Career Award from the National Fire Control Symposium, and was awarded a NASA Early Career Fellowship for increasing science autonomy in space.
Programe Committee
- Athirai A. Irissappane, University of Washington, US
- Adrian Agogino, University of California, Santa Cruz, US
- Raphael Avalos, Vrije Universiteit Brussel, BE
- Wolfram Barfuss, University of Leeds, UK
- Reinaldo Bianchi, FEI University Center, BR
- Daan Bloembergen, Centrum Wiskunde & Informatica, NL
- Rodrigo Bonini, Federal University of ABC, BR
- Roland Bouffanais, University of Ottawa, CA
- Vinicius Carvalho, University of São Paulo, BR
- Raphael Cobe, Advanced Institute for AI, BR
- Esther Colombini, University of Campinas, BR
- Anna Costa, University of São Paulo, BR
- Richard Dazeley, Deakin University, AU
- Mathijs De Weerdt, Delft University of Technology, NL
- Yunshu Du, Washington State University, US
- Elias Fernández Domingos, Vrije Universiteit Brussel, BE
- Cameron Foale, c.foale@federation.edu.au, AU
- Julian Garcia, Monash University, AU
- Ruben Glatt, Lawrence Livermore National Lab, US
- Josiah Hanna, University of Edinburgh, UK
- Brent Harrison, Georgia Institute of Technology, US
- Daniel Hernandez, University of York, UK
- Pablo Hernandez-Leal, Borealis AI, CA
- Michael Kaisers, Centrum Wiskunde & Informatica, NL
- Johan Källström, Linköping University, SE
- Mari Kawakatsu, Princeton University, US
- Ho-fung Leung, The Chinese University of Hong Kong, HK
- Guangliang Li, University of Amsterdam, NL
- Pieter Libin, Vrije Universiteit Brussel, BE
- Kleanthis Malialis, University of Cyprus, CY
- Karl Mason, Cardiff University, UK
- Ramona Merhej, INESC-ID and IST, University of Lisbon, PT
- Arno Moonens, Vrije Universiteit Brussel, BE
- Frans Oliehoek, Delft University of Technology, NL
- Bei Peng, University of Oxford, UK
- Hélène Plisnier, Vrije Universiteit Brussel, BE
- Gabriel Ramos, Universidade do Vale do Rio dos Sinos, BR
- Giorgia Ramponi, Politecnico di Milano, IT
- Mathieu Reymond, Vrije Universiteit Brussel, BE
- Golden Rockefeller, Oregon State University, US
- Francisco Santos, Universidade de Lisboa, PT
- Yash Satsangi, Tilburg University, NL
- Jivko Sinapov, Tufts University, US
- Ibrahim Sobh, Valeo, EG
- Denis Steckelmacher, Vrije Universiteit Brussel, BE
- Paolo Turrini, University of Warwick, UK
- Peter Vamplew, Federation University Australia, AU
- Miguel Vasco, INESC-ID and IST, University of Lisbon, PT
- Vitor Vasconcelos, University of Amsterdam & Princeton University, NL/US
- Timothy Verstraeten, Vrije Universiteit Brussel, BE
- Baoxiang Wang, The Chinese University of Hong Kong, HK
- Marco Wiering, University of Groningen, NL
- Connor Yates, Oregon State University, US
- Changxi Zhu, South China University of Technology, CN
- Luisa Zintgraf, University of Oxford, UK
Organization
This year's workshop is organised by:- Conor Hayes (National University of Ireland Galway, IE)
- Felipe Leno da Silva (Lawrence Livermore National Lab, US)
- Roxana Rădulescu (Vrije Universiteit Brussel, BE)
- Diederik M. Roijers (Vrije Universiteit Brussel, BE; HU University of Applied Science Utrecht, NL)
- Fernando P. Santos (Princeton University, US)
- Enda Howley (National University of Ireland Galway, IE)
- Daniel Kudenko (University of York, UK)
- Patrick Mannion (National University of Ireland Galway, IE)
- Ann Nowé (Vrije Universiteit Brussel, BE)
- Sandip Sen (University of Tulsa, US)
- Peter Stone (University of Texas at Austin, US)
- Matthew Taylor (Washington State University, US)
- Kagan Tumer (Oregon State University, US)
- Karl Tuyls (University of Liverpool, UK)
Sponsorship
The ALA 2021 Best Paper Award is kindly sponsored by the Springer journal Neural Computing and Applications.
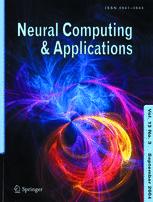